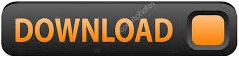

There are two ways to run this, one is running with the RAPIDS Accelerator set to explain only mode and the other is to modify your existing Spark application code to call a function directly. This allows running queries on the CPU and the RAPIDS Accelerator will evaluate the queries as if it was going to run on the GPU and tell you what would and wouldn’t have been run on the GPU. However it is very convenient because you can run the tools on existing logs and do not need a GPU cluster to run the tools. Since the two tools are only analyzing Spark event logs they do not have the detail that can be captured from a running Spark job. |appIndex|sqlID|nodeID|nodeName |accumulatorId|name |max_value |metricType| Those are indicators for a good candidate application for the RAPIDS Accelerator. In the following example the profiling tool output for a specific Spark application shows that it has a query with a large HashAggregate and SortMergeJoin.
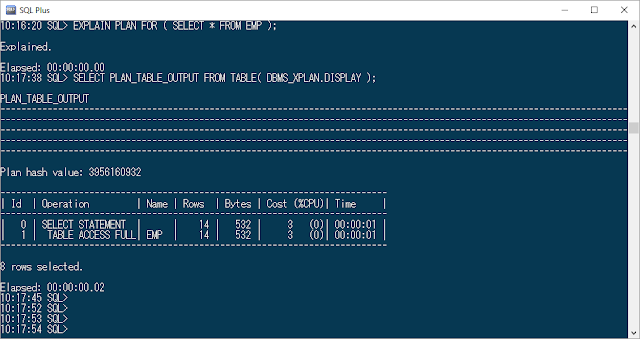
The profiling tool outputs SQL plan metrics and also prints out actual query plans to provide more insights. Its output can help you focus on the Spark applications which are best suited for the GPU. For example, the CSV output can print Unsupported Read File Formats and Types, Unsupported Write Data Format and Potential Problems which are the indication of some not-supported features. The Qualification tool outputs the score, rank and some of the potentially not-supported features for each Spark application. If you have Spark event logs from prior runs of the applications on Spark 2.x or 3.x, you can use the Qualification tool and Profiling tool to analyze them. Qualification and Profiling tool Requirements
XPLAN RECOMMENDED GPU HOW TO
This article describes the tools we provide and how to do gap analysis and workload qualification. It all depends on how critical the portion that is executing on the CPU is to the overall performance of the query. Significant performance benefits can be gained even if all operations are not yet fully supported by the GPU. Understanding this will help prioritize workloads that are best suited to the GPU. When converting an existing Spark workload from CPU to GPU, it is recommended to do an analysis to understand if there are any features (functions, expressions, data types, data formats) that do not yet run on the GPU. There may be some performance overhead because of host memory to GPU memory transfer. If there are operators which do not yet run on GPU, they will seamlessly fallback to the CPU. The RAPIDS Accelerator for Apache Spark runs as many operations as possible on the GPU. Getting Started on Spark workload qualification
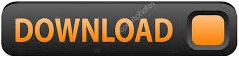